Machine learning (ML) might seem intimidating at first, but with the right guidance, you can quickly grasp its core concepts and start building your own models. Whether you're a data enthusiast or someone looking to dive into AI, this step-by-step guide will walk you through the process of creating your first machine learning model.
By the end of this guide, you’ll have a basic ML model up and running. Let’s get started!
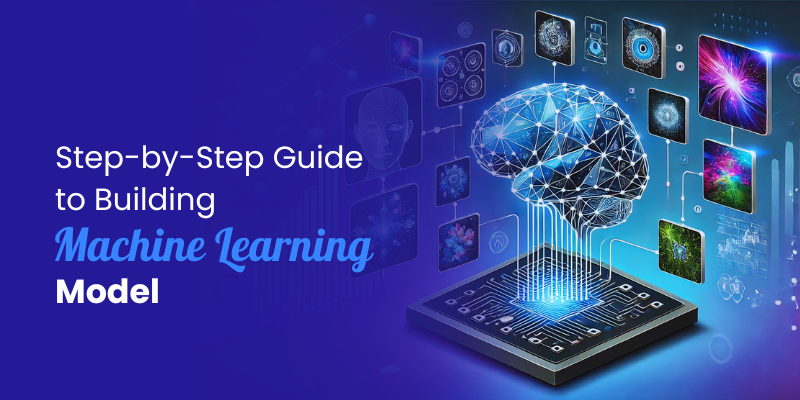
Step 1: Define the Problem
The first step in building any machine learning model is defining the problem you’re trying to solve. Are you looking to predict values, classify data, or find hidden patterns?
For example, let’s say we want to predict the prices of houses based on features such as size, location, and number of rooms. This would be a regression problem because we're predicting continuous values (prices).
For classification problems, the goal might be to classify emails as “spam” or “not spam” based on certain features (e.g., keywords in the subject line).
Step 2: Collect and Prepare the Data
Once you've defined the problem, you need data to train your machine learning model. Data is the foundation of any machine learning project, so it’s crucial to have relevant and high-quality data.
Where to find data:
Kaggle (a platform with tons of datasets for various ML tasks)
UCI Machine Learning Repository
Public datasets on GitHub
For our house price prediction example, you might use a dataset that includes various features like square footage, neighborhood, number of rooms, and price.
Once you have your dataset, you’ll need to clean and preprocess it. This involves:
Handling missing values: Replace or remove missing data points.
Feature scaling: Normalize or standardize numerical features to ensure consistency across the data.
Encoding categorical variables: Convert categorical data (e.g., "red," "blue") into numerical values using techniques like one-hot encoding.
Step 3: Split the Data
Before you start training your machine learning model, it’s essential to split your data into two parts:
Training Data: Used to train the model (typically 70-80% of the dataset).
Test Data: Used to evaluate the model's performance after training (usually 20-30% of the dataset).
This step is crucial because it allows you to check how well your model generalizes to unseen data. If you use all the data for training, your model might overfit and perform poorly on new data.
Step 4: Choose a Machine Learning Algorithm
There are various machine learning algorithms, each suited for different types of problems. For beginners, we recommend starting with simple algorithms that are easy to understand and implement.
For regression problems:
Linear Regression: This algorithm fits a straight line to the data and predicts continuous values. It’s a great starting point for predicting house prices.
For classification problems:
Logistic Regression: Despite its name, it’s a classification algorithm that is commonly used for binary classification (e.g., spam or not spam).
K-Nearest Neighbors (KNN): This algorithm classifies data points based on the closest neighbors in the feature space.
If you're using Python, you can easily implement these algorithms using libraries like scikit-learn.
Step 5: Train the Model
Now comes the exciting part—training your machine learning model! Using your training data, you’ll train the model to learn patterns in the data.
For example, in Python, you might write the following code to train a simple linear regression model using scikit-learn:
python
Copy code
from sklearn.model_selection import train_test_split from sklearn.linear_model import LinearRegression # Split data into features (X) and target (y) X = dataset[['Square_Feet', 'Num_Rooms', 'Location']] # Example features y = dataset['Price'] # Target variable (Price) # Split the dataset into training and test sets X_train, X_test, y_train, y_test = train_test_split(X, y, test_size=0.2) # Create a Linear Regression model model = LinearRegression() # Train the model using the training data model.fit(X_train, y_train)
In this code, we:
Split the data into features (X) and the target variable (y).
Split the data further into training and testing sets.
Create a model object (linear regression in this case) and train it using the training data.
Step 6: Evaluate the Model
Once your model is trained, it’s time to evaluate its performance on the test data. This will give you an idea of how well your model generalizes to new, unseen data.
You can use various metrics to evaluate the model, depending on the type of problem you’re solving.
For regression problems, common evaluation metrics include:
Mean Absolute Error (MAE): The average of the absolute differences between predicted and actual values.
Mean Squared Error (MSE): The average of the squared differences between predicted and actual values.
R-squared: A statistical measure that indicates how well the model’s predictions match the actual data.
For classification problems, you can use:
Accuracy: The percentage of correctly predicted instances.
Precision and Recall: Metrics for evaluating performance when classes are imbalanced.
Here’s an example of evaluating the model’s performance in Python:
python
Copy code
from sklearn.metrics import mean_squared_error, r2_score # Predict the target values on the test set y_pred = model.predict(X_test) # Calculate Mean Squared Error mse = mean_squared_error(y_test, y_pred) # Calculate R-squared value r2 = r2_score(y_test, y_pred) print("Mean Squared Error:", mse) print("R-squared:", r2)
Step 7: Improve the Model
After evaluating the model, you might find that it needs improvement. There are several ways to enhance the performance of your model:
Tune hyperparameters: Adjust the settings (hyperparameters) of your algorithm to find the best combination for your data.
Feature engineering: Create new features or modify existing ones to better represent the data.
Use more advanced algorithms: If the simpler models aren’t performing well, consider trying more complex models like decision trees, random forests, or support vector machines.
Step 8: Make Predictions
Once you’re satisfied with the performance of your model, you can use it to make predictions on new data. For instance, with the house price prediction model, you can now predict prices for houses with different features.
Here’s how you’d make predictions:
python
Copy code
new_data = [[2500, 3, 'Downtown']] # Example new data price_prediction = model.predict(new_data) print("Predicted House Price:", price_prediction)
Step 9: Deploy the Model
The final step is to deploy your model so it can start making real-time predictions. Depending on the application, you might integrate the model into a web application, mobile app, or business tool.
Tools like Flask, FastAPI, or cloud services like AWS SageMaker and Google Cloud AI can help you deploy your model.
Conclusion
Building your first machine learning model can be challenging, but by following these steps, you’ll be well on your way to understanding and creating ML models. The more you practice, the better you’ll get at tweaking models, optimizing performance, and solving real-world problems.
Start small, experiment with different algorithms, and, most importantly, have fun with the process. Machine learning is a powerful skill that can unlock endless possibilities in fields like data science, artificial intelligence, and beyond!
Ready to dive in? Start with a simple dataset and follow these steps to build your own first machine learning model! 🌟
You can check more info about: Impact of Data Engineering on Business Intelligence and Analytics.
Kommentare